Manufacturers Leverage IIoT Data
/As companies make digital transformations using IIoT data, they see immediate results that save time and money.
By Michelle Segrest, Navigate Content, Inc. - Reporting for Efficient Plant Magazine
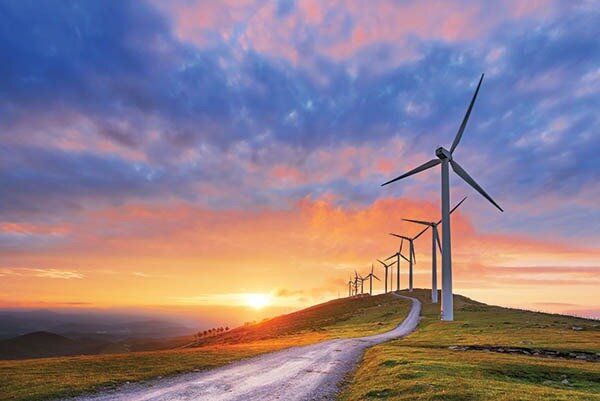
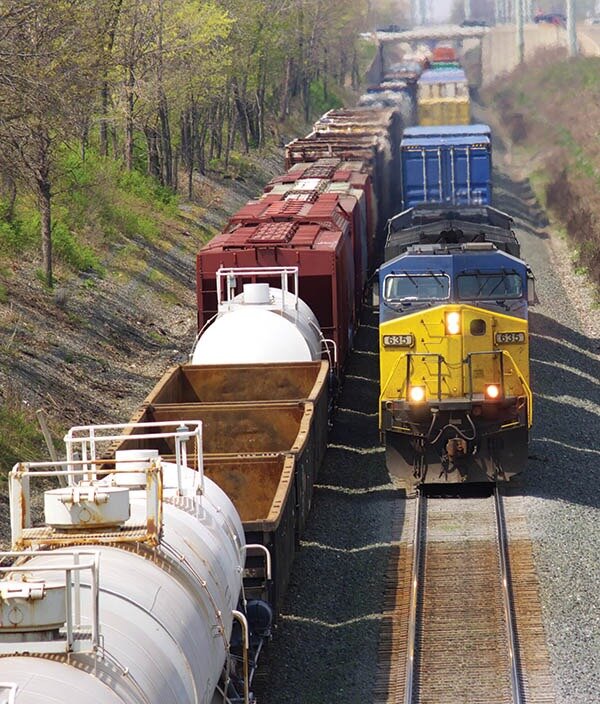
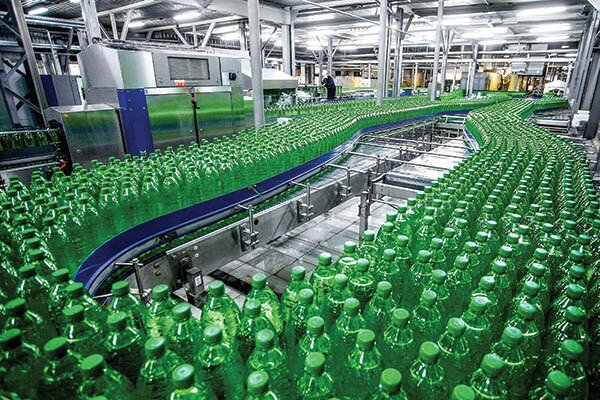
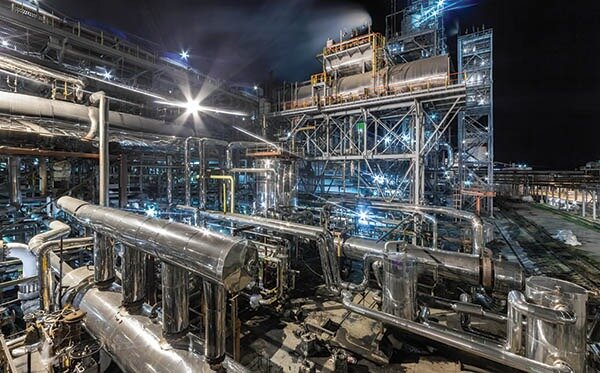
A plastics manufacturer had a major piece of equipment that was frequently breaking down with no warning. It was a $40-million problem every time it happened. By incorporating a digital-transformation strategy, the company was able to predict the problem five days in advance.
“This is the power of the Industrial Internet of Things (IIoT),” said Robert Golightly, senior manager, Product Manufacturing at AspenTech, Bedford, MA (aspentech.com), a supplier of software that optimizes asset performance. “When a machine fails, companies generally wait until the machine is fixed, and when the process is running again, they go back to work. They are forced to do this because there is not enough time to do anything else. But when you have a few more days or even weeks to think about it, suddenly there are all kinds of options. You can use the time to build inventory or reschedule production. There are half-dozen smart business decisions we could make if we had enough time.”
IIoT is having a profound effect on the way companies collect, manage, and analyze data.
Siemens is a global-technology company that is springing into action to provide digital-technology strategies. Siemens has spent more than $10 billion to acquire software companies in the past 10 years and employs more than 24,500 software engineers.
“IIoT is not a silver bullet for everything,” said Alistair Orchard, vice president, Digital Enterprise, Siemens. “It’s not the entire identity of our digitalization platform, but it is highly central through our strategy. We complement IoT with things like simulation, augmented reality, and digital-twin technology.”
More than 23,000 companies offer IIoT solutions worldwide. To weed through them effectively, Orchard recommends asking five critical questions:
Connectivity: Does the IIoT platform retrieve data from as many sources as possible?
Ecosystem: Do enough people use it to make it viable?
Scalability: Will the platform deliver solutions that can work throughout your plant and throughout other plants in your company?
App Ecosystem: If you have to build all of your own apps it will be expensive, difficult, and time consuming. Does the IIoT platform offer an app store or the ability to build apps specific to your needs?
Subscriptions: Does the company have a program that allows you to test a platform on a subscription basis to be sure it fits your needs? “This is a big game changer for companies,” Orchard said. “Previously, not only was it expensive to collect data, but you also had to purchase many licenses. It was a major commitment. Now you can rent a program for a month, engage with consultants, take workshops to identify use cases, and begin downloading or building apps to solve problems. If, after a month, you’re not happy and not receiving value, you can just switch it off. If you like it, you can quickly scale and apply the same algorithms to all your factories. You can start small with no commitment.”
Data-Analysis Makes a Difference in Manufacturing
Aspentech’s Golightly said there are key things about digital-transformation strategies that have changed the face of manufacturing. “IIoT can be an effective way for us to tear down silos and integrate our work flows,” he explained. “It’s clear that the way we sense that something is wrong, how we classify it, how we determine a strategy to deal with it, and the way we execute that strategy is highly variable across companies, locations, cultures, and lines of business. I think the big brass ring with IIoT is that it gives us a way to work better together and helps us make big decisions with more information.”
Low-touch machine learning is an element of IIoT that is closing the industry’s talent gap, Golightly said. “You may do a lot of reading about deep learning, machine learning, and artificial intelligence. Somewhere in the course of that discourse there will be some reference to a data lake and some reference to a team of data scientists.
There are only about 150,000 of those guys out there, and we are all trying to hire them. First, they get their information in place, then they hire their data-science team, then they get the latest toys. It takes several years before they earn their keep. We are not trying to build a data-science platform. We are trying to use machine learning to solve one big, very particular problem—‘Tell me as soon as you can when a machine is going to break.’”
Many types of data can be collected in many ways, but the elements must be strung together to create anything useful, according to Orchard. The key challenge becomes weeding out the bad data and then using the good data to make a difference in plant efficiency.
“One of the great things about IIoT is you can collect a lot of useful data at a minimal cost,” Orchard explained. “You can easily see what’s going on in a facility by gluing a little $10 plastic sensor to your equipment. It can detect vibration and other problems. You can place IoT sensors everywhere. They become part of the infrastructure and communicate with software throughout the plant. It is an inexpensive way of recognizing temperature, vibration, location, and current, among other things.”
There are about a million devices connected into Siemen’s Mindsphere platform, which is a cloud-based, open IoT operating system that connects products, plants, systems, and machines, enabling companies to harness the wealth of data generated by IoT with advanced analytics.
“Our initial focus was going in and grabbing data from the easy places,” Orchard said. “The second part of our infrastructure is cloud based where we stream the data. This centralizes the data and makes it available. You’d be surprised how excited people get just seeing what’s going on—things that are not traditionally visible outside a machine. It’s impactful just being able to quickly see it from a central location and comparing raw data from across the plant. It’s simple stuff, but you look at the customers’ faces, and you can see they consider it relatively miraculous.”
Siemens also incorporates a series of management apps that can define the information more intensely. Apps can be created specifically for customers, or customers can build their own, or they can choose from others already available within the Mindsphere app store.
“The most interesting are the machine-learning and artificial-intelligence plugins available that enable us to do more collaborative things with the data,” Orchard said. “We can visualize the data and start to predict what’s going on. The obvious first thing that customers are interested in is predictive maintenance.”
Companies can now use the information to look beyond the horizon to understand downstream implications of their decisions. “At the systems level you are trying to answer that big question, ‘If this breaks, what happens?’” Golightly said. “This is critical intel. Its function is to use machine learning and look at real-time process data to help us know weeks or even months in advance that a machine is going to break so we can do smarter things to maybe avoid the downtime altogether. When you can solve those problems in advance, it produces compelling payback.”
Real-World Examples of Manufacturers Levering IIoT Data
Saras S.p.A., Sarroch, Italy (saras.it), owner of the most complex refinery in the Mediterranean, has a capacity of 300,000 barrels/day. It uses Aspen Mtell software to drive reliability in its capital- and asset-intensive refining operations, particularly for critical equipment such as large compressors and pumps. Leveraging this software, Saras received detection accuracy of 91% with 30 days of lead time.
“Improving reliability positively impacts a wide range of issues, from reducing current maintenance costs to planning for abnormal process conditions, avoiding emergency or unplanned shutdowns, and successfully managing unpredictable feed and demands,” said Alessandro Zucca, digital platform manager, Operations and Assets, Saras.
Siemens worked with a soft-drink bottling plant that uses hundreds of motors. It bottles products quickly—thousands every minute. The plant’s mostly ancient motors fail occasionally and don’t have any analytical capabilities built in, making it difficult to preventively maintain them.
“They [plant personnel] discovered over time that it is better to just wait for something to break, but they get occasional disastrous failures,” Orchard said. “This means they must keep spare parts in the plant to get things working quickly again.”
Siemens placed a $10 sensor on each of the motors at a total cost of around $3,000. They were installed in one day and operate on Bluetooth internally in the factory. “We can use the sensors to monitor vibration and predict failure,” Orchard said. “The plant [personnel] can now centralize all the maintenance on multiple motors and replace them before they break down.”
Vibration information is sent into the Mindsphere platform, where an artificial-intelligence (AI) technique begins to strip away the noise and identify the point of vibration within the specific motor. The points of vibration are matched with the predicted failure. “It [Mindsphere] can tell you that within the next couple weeks you will have bearing failure, so you should swap those now during an already scheduled shutdown,” he explained.
The vibration data is extremely high resolution and provides 6,300 points of detailed data that identify the problem. Devices on the shop floor receive the data from the sensors, then pre-process and clean the data with only the relevant data sent to the cloud.
“What you’ll find with most IIoT platforms is that, in order to apply the clever artificial-intelligence and machine-learning algorithms (the ones that do prediction), you need two types of people,” Orchard explained. “You need a data scientist. They are brainy and expensive and there are not many of them, but they know nothing about motors. Then you need a motor expert. These two people have different kinds of brains and different vocabularies. You can’t apply the algorithms until you understand them. The motor expert understands the equipment but may not know anything about analytics. It’s important to get these two types of people talking to each other. This makes the whole process expensive. If I have different types of motors, I need a data scientist for each one and a different expert for each one. This is one of the drawbacks of this type of application.”
Here is where digital-twin technology comes into play. A digital twin—in this case, a virtual model of the motor—can simulate the process. “When we are designing the motors, we use sophisticated simulation to predict things like speed, torque, temperature, and vibration. This is all possible during the design stage of the motor,” Orchard said. “We imbed the model into our IoT platform. We can then send the IoT data from the shop floor and compare what we predicted in our highly sophisticated simulation to what’s actually happening on the shop floor. You don’t need a data scientist or a motor expert because the expertise is built into the digital twin. This makes data science easy because you are comparing predicted trends to actual trends. This closes the loop between predicted analytics and actual IIoT.”
Borealis, a Vienna, Austria-based provider of innovative solutions in the fields of polyolefins, base chemicals, and fertilizers, used Aspen Mtell software to provide longer lead-time detection of repeating failures, including 27 days of advanced notice for central-valve failure, transfer learning, and the ability to capture fast-moving failures at its production site in Stenungsund, Sweden. Borealis leverages the software to mine historical and real-time operational and maintenance data to discover precise failure signatures that precede asset degradation and breakdowns, predict future failures, and prescribe detailed actions to mitigate or solve problems.
“Aspen Mtell predictive-maintenance software’s ease of implementation allows us to develop data analytics, including pattern recognition and early anomaly detection in all operating functions, leading to increased performance in safety, quality, reliability, and overall improved performance in manufacturing,” said Martijn van Koten, executive vice president, Operations, Borealis.
Since applying its own IIoT data strategies and techniques in the Siemens Electronic Works plant in Amberg, Germany, the company has increased the efficiency of its operations by a factor of 13. “We managed to achieve an overall quality of 99.9999%,” Orchard said. “The most important thing for us in that factory is quality. Through careful planning and quality processes and by adding sensors to the equipment, we have managed to get to the point where our biggest quality issue is with the raw materials that we bring in. We make a million products in that facility every month; that’s one every second. We need hundreds of millions of parts. The quality of the parts is lower than the quality of our final product. This is magic. We were able to improve all the quality issues within the process.”
Orchard explained that there are two ways to do this. “You can test every component within the company, but this is literally impossible,” he said. “Because we make one product per second, we would have to slow the production down and x-ray the product to identify and electronically test every single product. Instead, we collect hundreds of points of complex data from the shop floor. There are 40 dimensions to the data, all very interrelated in complex ways. When you look at this data, it’s impossible for a human to understand whether the data is good or bad. We feed all the data into Mindsphere, then apply machine learning to refine it to a point where we can use it effectively.”
Orchard explained machine learning with a simple example. “When you go into Google and search for ‘cat,’ it brings up a whole bunch of images,” he said. “No one actually sat down and went through the billions of photos and tagged every single one of them as ‘cat.’ It was done through machine learning. Google started feeding data into the computer, identifying a few species of cat and identifying others as ‘not a cat.’ After a while, the computer takes over and begins to understand what is and what isn’t a cat. It’s really clever. It can understand big cats, small cats, gray cats, tabby cats. You don’t have to tell it. This is called supervised machine learning.”
This is the technique Siemens used at its Amberg facility. “We fed it nine-million records, nine months of production data, and we used machine learning in Mindsphere to build its algorithm,” Orchard explained. “Now, once every second, Mindsphere determines instantly whether a product is good or bad without any x-raying or electronic testing or final product testing. That’s how we use IIoT to make extremely sophisticated analyses. This increases our throughput while producing almost perfect quality.”
Siemens also performed an energy project at the Amberg facility using IoT data. In the first two weeks of the project Siemens saved about 20,000 Euros per month in energy, saved 30,000 euros in nitrogen, and reduced compressed-air usage by 12%.
CSX Corp., a transportation-services provider based in Jacksonville, Florida saved millions of dollars in costly downtime by using AspenTech’s Mtell for prescriptive-maintenance solutions. The prescriptive-maintenance functionality helps CSX identify potential locomotive issues by analyzing large volumes of real-time and historical operational and maintenance data to detect anomalous behavioral patterns. By automating the detection of potential problems and prescribing corrective actions, the solution helps firms quickly identify and rapidly respond to early indicators of potential failures, significantly improving asset utilization.
Siemens uses its IIoT sensors for huge wind turbine parks worldwide. “If you want to maintain these giant wind turbines, just climbing onto them is a dangerous and difficult feat,” Orchard said. “Also, they are generally in the middle of nowhere; middle of the sea, or on a mountain, or in the desert. It’s important to remotely monitor them. We add IoT sensors to the windmills, then use Mindsphere to visualize what’s going on and to perform predictive maintenance.”
This increases safety and uptime, while optimizing operation. “We take it one step further by using our digital-twin technique,” he added. “It’s expensive to install all the sensors you need to fully monitor one of these wind turbines. We use a digital twin of the entire wind turbine and cover it with virtual sensors. On the real wind turbine, we install one sensor, a sophisticated gyroscope. We stream this sensor data into Mindsphere and then send the digital data into the digital twin. That twin can then predict vibration, cracks in the column, overheating bearing problems, and foundation issues.”
Tips to Improve Manufacturing Efficiency
The challenges within manufacturing haven’t changed through the years, according to Orchard. “Everyone wants more transparency. Everyone wants more uptime. Everyone wants more efficiency. Everyone wants proper diagnoses and resolution of problems. This has all been traditionally very expensive. IIoT allows us to do all of this at a very low price point and in a manner which scales quickly across an entire manufacturing footprint. It hasn’t changed the goals of our customers. It’s changed the speed and cost and efficiency of doing those things.”
Asking the right questions is where any company should begin, according to Golightly. “The one thing, and really the only thing, that I think separates the real analyst from someone who is faking it is they know how to ask the right questions,” he said. “The rest of it is straightforward. I think the struggles we have in determining what data you need and how to condition it concern formulation of the right questions.”
Golightly said it’s a decomposition process. “You need to find insight to create the business value,” he said. “This drives the alignment and the collaboration that needs to happen upfront before you [the end user] start getting your hands dirty in data. We must ask the right questions in the right context and make sure that the organization is ready and willing to do something with the insights you deliver. If not, then we are all guilty of just wasting a lot of time.”
Collecting the data and analyzing it are only the first steps. The key is taking action.
“We’ve gotten past the point in our evolution where the Lone Ranger is going to go out there and grab the data and solve the problem for us,” Golightly said. “We probably don’t realize the degree to which collaboration is an essential ingredient in all of this. That is the secret sauce.”
Golightly said there are no limits to the value of using IIoT in daily operations. “We are at a remarkable point,” he stated. “The power that we have as individuals and teams is almost incomprehensible. I’m as excited as I’ve ever been watching people solve problems that we’ve struggled with for decades. This is a fantastic time to be in this game. These new creative minds will take the new capabilities and use them in ways that we haven’t thought of. We need to get out of their way and let them do that.”
Michelle Segrest is president of Navigate Content Inc., and has been a professional journalist for three decades. She specializes in creating content for the processing industries. If you have an efficiency, maintenance, and/or reliability story, contact her at michelle@navigatecontent.com.
If you like this article about how manufacturers leverage IIoT, please PIN IT!
This page contains affiliate links. If you click on the product links and make a purchase, it allows me to make a small commission at no extra cost to you! Thank you for your support and I hope you find value in this content!